Category: Research Data Management (RDM)
Data Management Plan
Data Management Plan (DMP)
Writing a data management plan (DMP) when you start your research can help to ensure you achieve a standard of transparency and integrity in your research. In a DMP you describe the data you plan to collect, generate and use for your research, whether it is data you create yourself of existing data created by someone else. You describe in a structured way how you will manage those data during and after your research. You write about:
- how the data were created and what they mean
- safe storage of data so they can’t go missing or be tampered with
- data security so that only people allowed to access the data can access them
- publishing the data as evidence for your published papers (unless there are restrictions to do so), so that your findings can be verified and reproduced
- individual and institutional responsibilities to look after your data
- safeguards for ethical and privacy reasons if research involves human participants
Good data management does not end with planning. It is important that your research data are then managed according to this plan. You can review and update the plan according to the progress of the research (it’s a living document). After your research has ended your DMP will form the permanent track record for the data your research produced.
Key issues to find out when you start writing a data management plan are:
- know your institution’s policies and services, such as storage and backup strategy, intellectual property rights policy, data management policy and any data sharing facilities like an institutional repository
- ownership of your data
- your legal, ethical and other obligations regarding research data, towards research participants, colleagues, research funders and your institution
Here are some examples where good data management plans can help achieve research integrity:
- Documenting in detail how your data were created and processed provides clear evidence, for example:
- a lab book describes the experimental set up and all parameters that define your data, show the processes used to collect the data and gives and overview of all data collected
- an interview schedule and question list describe the collection of information via interviews, with a referenced codebook showing your interpretation of the interview content during your analysis
- commentary lines in computer code describe the logic of what your code does step by step.
- The licence agreement and use conditions of third party data explain how you can or cannot use third party data in your research, for example you may be allowed to use data for analysis but not copy and publish them ad you should cite the data you use (like you would cite a publication) whereby it is important to check the ownership of those data.
- In research with human participants, documenting the informed consent procedures used in your research and the personal data you may collect helps to plan which level of security is needed when storing and handling the data and how data need to be anonymised to respect the privacy of people.
- Publishing your research data in a FAIR way gives transparency about how you reached your research findings bases on those data.
- If other researchers want to reproduce your results, they need to be able to access the data and any documentation that clearly explains how the data were generated and how to interpret them. Data can for example be made available openly in a data repository with a clear use licence.
In collaborative research, it is important to describe the planned data management practices at each partner organisation, and to have a dedicated person at each site responsible for data management. In international collaborations, it can be that there are differences in the ethical and legal framework for research, or the expectation of institutions or funders for data management. Developing a data management plan can help to ensure that all these aspects are addressed before data collection starts.
FAIR data principles for research data
FAIR data principles for research data
One of the main objectives of a good RDM practice during the research project is to enable the long-term preservation of FAIR data objects after the research results have been published and/or the research project has ended. By preserving data for the long-term, it becomes possible to (1) reproduce the findings of a certain study at a later stage and to (2) re-use the data for new research purposes.
The guiding principle should be that the data are as open as possible, and as closed as necessary.
Long-term preservation of the data in itself is not sufficient to turn the data into a valuable and citable research output that is on par with publications. Barely documented data can be stored for a very, very long time on the private server of a research department, gathering dust and steadily sinking into oblivion. But digital innovations will leave that data unreadable for both machine and researcher.
Detailed documentation of you data collection processes (as part of your general data management) can help to ensure that any selection of dates clear, readable and can be made available for others. Also preregistration of your methodology cam help to prevent any threat research integrity.
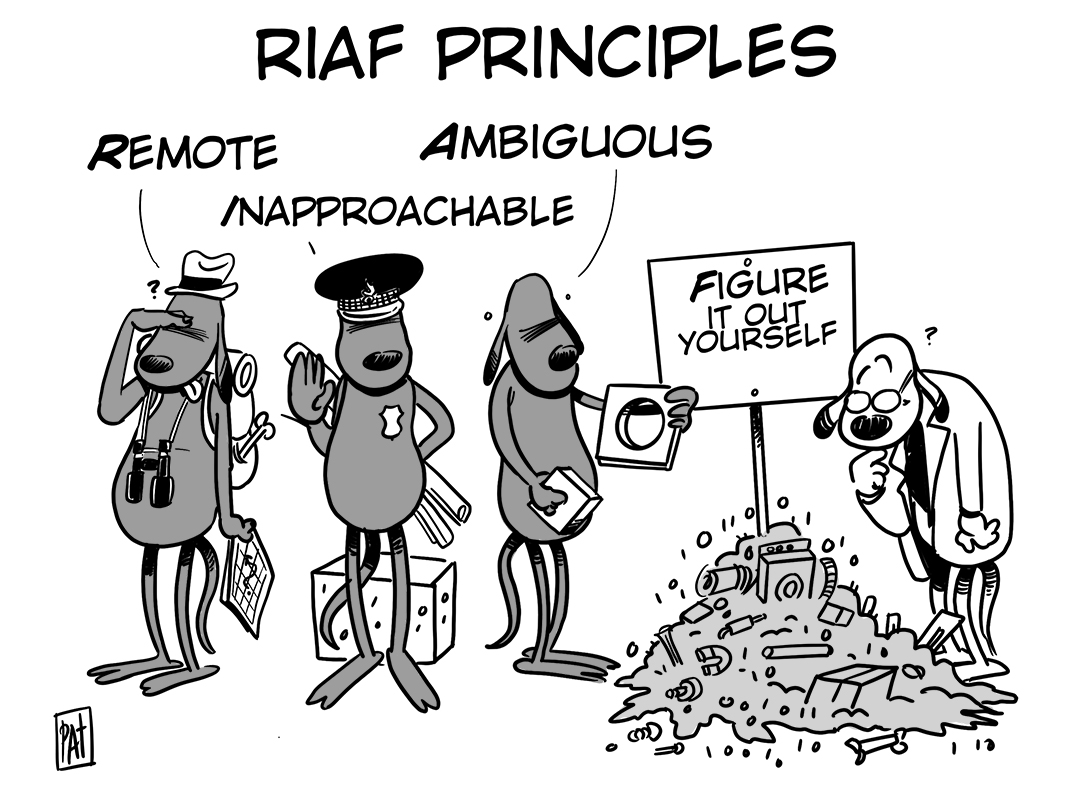
Cartoon by Patrick Hochstenbach under a Creative Commons CC BY-SA 4.0 license
FAIR Principles
This is where the FAIR guiding principles for scientific data management and stewardship enter the picture. The FAIR principles were initially conceived for research data (Wilkinson et al, 2016), but are also being applied to more specific types of research outputs such as software (Lamprecht et al, 2019). Data can be turned into FAIR objects, which makes them ‘exploitable’ for the broader research community in the long run. FAIR stands for Findable, Accessible, Interoperable and Re-usable.
- Findable
Ideally, data and accompanying documentation/metadata are made findable by both humans and ICT systems. Concretely, this findability is typically ensured by means of ‘discovery metadata’ that are available via a data search engine such as DataCite. If you search for the data on the search engine, the associated discovery metadata, including the name(s) of the data creator(s), the subject of the data etc., pop up among the search results. Commonly, the discovery metadata would include a persistent identifier (e.g. DOI, handle, etc.) that directs you to the landing page where the (non-sensitive) data are available for download. Note that findability comes in different flavors. Data published on a personal website or a specific project website are to some extent findable, but not in any meaningful, structural way. - Accessible
The access conditions for the data are well-defined, supported by the appropriate license (e.g. a Creative Commons license for open data). Data are published in open access when possible, but restricted/closed access is applied in case of sensitive data (e.g. personal data). Although sensitive data are not publicly made available in open access, they can still often by re-used by other researchers, albeit via a more complex procedure that safeguards the rights of the data subjects and ensures data security. Note that the discovery metadata referring to the sensitive data can still be publicly available, even though the data themselves are not. - Interoperable
Interoperable data are data that can be combined with other datasets by humans as well as ICT systems, and has no unnecessary legal obstacles (e.g. OA license with overly complex restrictions). Additionally, the data can easily interoperate with automatised analysis workflows or other applications. It is also important that the documentation/metadata accompanying the data maximally adhere to discipline-specific standards, for instance by using ‘controlled vocabularies’ and can be encoded in a standardised, structured format in order to make them machine-readable. Examples of generic metadata standards are the Dublin Core and DataCite Metadata Schema. - Re-usable
The three pillars ‘findable’, ‘accessible’ and ‘interoperable’ are all necessary prerequisites to make the data eventually re-usable and interpretable by other researchers. Particularly important is the documentation/metadata that accompanies the data such as, f.e. a codebook that explains the different variables or an explanation of how the data were collected. Without the adequate documentation, data are generally difficult to interpret, which obviously hampers re-use. Note that, if the data are sensitive, re-use is not impossible, but has to comply with stringent conditions stipulated in a ‘data use agreement’.
Data Repositories
Researchers should hone their FAIRification skills, in order to make the data that they collect or generate as FAIR as possible. However, they are not alone in this endeavour. Next to supporting RDM services at research institutions, there is also a pivotal role for the ‘trustworthy repository’ where the research data are ultimately archived. For example, the data repository can be well-connected to the broader data ecosystem, enhancing the findability of the archived data, and provide infrastructure to implement certain metadata standards, improving interoperability.
- Re3data is a registry of institutional, disciplinary and interdisciplinary research data repositories worldwide.
When to think about this?
As already stated before: “RDM includes all steps before, during and after the project” which means you have to handle your research data correctly throughout the whole research data lifecyle to ensure the quality and integrity of your research. A data management plan (DMP) is the best tool to help you to do just that.
Research Data Management (RDM)
Research Data Management (RDM)
What are ‘research data’?
Research data are all digital or physical data – regardless of the manner in which these data are collected or stored – used or analysed to support research findings, validate research results or support a scientific reasoning, discussion or calculation in the study. Research data cover the entire spectrum from raw data to processed and analysed data included or discussed in a publication. These data can be generated data, derived or composite data, as well as self-generated data and data provided by third parties. Some examples are: survey results, statistical data, graphics, computer-generated data, simulations, software developed for research purposes, computational metadata, prints, video and audio tapes, a corpus, organisms, gene sequences, synthetic or chemical compounds, samples of any kind, patient records, protocols, measurements, notebooks, and so on.
Research data form the beating heart of academic research, and are the engine of enormous progress in technology, healthcare and on a socio-economic level.
Research Data Management (RDM)
Although researchers are continuously working with data to test them against the research hypothesis, these are often not processed and stored carefully during and after the project. At the same time, our society is becoming ever more focused on increasing datafication, with more and more processes and actions based on digital data. This also enables a specific type of data-driven research, based on the combination of (big) data with new techniques for analysis (i.e. “data science”).
This large use and potential reuse of data therefore makes solid management of research data very important in the research community, meaning that the good handling of data, the so-called research data management (RDM), should be one of the cornerstones of good academic research practice.
RDM includes all steps before, during and after the project, i.e. “the research data lifecycle”: data planning, collection, processing, analysis, security, storage, preservation, access, sharing and reuse. All these steps are bound by conditions and regulations at legal, ethical and technological levels.
The importance of research data management
Prudent and thoughtful RDM leads to a better quality and integrity of research, a greater impact research and a greater visibility and reuse of research data. Good data handling can prevent data loss/corruption, fraud and/or bad science, it makes your research process run smoother, and ensures that you can find and reuse data later. In addition, the sharing and reuse of data for future researchers is facilitated in order to develop new research both inside and outside the university.
Imagine that you have read a scientific paper and would like to know how the algorithm the authors used works ‘under the hood’ or how the authors constructed a certain plot? How easy would it be if you could just click on a link and download that information? Imagine that you have to come up with a brilliant new way of analysing the data that underpin a certain study, but the original data are nowhere to be found. How much time would you lose collecting new data? Or imagine that you actually were able to get a hand on the original data, but after close scrutiny, the data are utterly incomprehensible: the measurement unit of variable B is impossible to decode etc.. How disappointed would you be after the initial effort of obtaining the data? Indeed, it’s such a hassle.
Every researcher has a responsibility to contribute to a new world where research data that underly research findings are easily findable and interpretable. Hence the need for proper research data management and researchers are increasingly required to put a thorough data management approach into practice throughout the research process. These fairly recent RDM requirements are often formalised in policies of research institutions, funders (e.g. the necessity to develop a data management plan) and journals (e.g. the inclusion of data availability statement in the article). At first glance, these RDM requirements might seem difficult to comply with for researchers who are not yet familiar with them. when you look further, you will notice that RDM will be of help during your research and with that they also serve the purpose of improving the way we do science, making scientific results more transparent and reproducible.
Retraction of an article because access to the underlying data was not granted.
Case: Lancet, NEJM retracts controversial COVID-19 studies based on surgisphere data – Retraction watch: “Two days after issuing expressions of concern about controversial papers on Covid-19, The Lancet and the New England Journal of Medicine have retracted the controversial articles on Covid-19 because a number of the authors were not granted access to the underlying data […]”.
“[…] Because all the authors were not granted access to the raw data and the raw data could not be made available to a third-party auditor, we are unable to validate the primary data sources underlying our article, “Cardiovascular Disease, Drug Therapy, and Mortality in Covid-19.” We therefore request that the article be retracted. We apologize to the editors and to readers of the journal for the difficulties that this has caused.””
Who is involved?
The researcher develops a data management plan for his/her research that describes how research data will be collected, organised, documented, stored, used and looked after throughout the research lifecycle; implements good data management practices; and ensures that the data remain available in the long term.
The supervisor supports and advises the researcher on data management practices and ethical, legal and contractual responsibilities.
The institution/university provides the tools, infrastructure and policies for the researcher to implement good data management practices.
Data stewards and research support staff provide advice, support and training on data management (planning) to the researcher.
The ALLEA Code also strongly confirms the importance of research data management and included the following:
- Researchers, research institutions, and organisations ensure appropriate stewardship, curation, and preservation of all data, metadata, protocols, code, software, and other research materials for a reasonable and clearly stated period.
- Researchers, research institutions, and organisations ensure that access to data is as open as possible, as closed as necessary, and where appropriate in line with the FAIR principles (Findable, Accessible, Interoperable and Reusable) for data management.
- Researchers, research institutions, and organisations are transparent about how to access and gain permission to use data, metadata, protocols, code, software, and other research materials.
- Researchers inform research participants about how their data will be used, reused, accessed, stored, and deleted, in compliance with GDPR.
- Researchers, research institutions, and organisations acknowledge data, metadata, protocols, code, software, and other research materials as legitimate and citable products of research.
- Researchers, research institutions, and organisations ensure that any contracts or agreements relating to research results include equitable and fair provisions for the management of their use, ownership, and protection under intellectual property rights.